By Gavin Duley
Wine quality and character depends in part on the environment the grapes are grown in, referred to as ‘terroir’: “Wine, according to its terroir, will have a particular taste that is associated with the soil, geology, topography, and climate where the vineyard is located” (Barnard 2016, pp. 14–15). This means the environment can influence the metabolome (metabolic patterns) of a wine. “Wine can be described as a molecular kaleidoscope integrating signatures from grapes, yeasts, the winemaking and the environment” (Roullier-Gall et al. 2014, p. 100).
This is interesting for two reasons. Firstly, chemical studies can demonstrate the reality of any terroir effects by providing objective proof of differences between the wines, e.g., the characterisation of appellations. Secondly, it can provide a method to detect wine fraud, especially misrepresentation of wine from a less prestigious region as being from a more prestigious region.
Many previous studies have been undertaken using metabolomic analysis to classify wines via region, vintage, and grape variety (Pereira et al. 2018; Wu et al. 2019), but few have utilised the latest technologies. In Australia, studies have employed HPLC and UV chemiluminescence (Bellomarino et al. 2009), ICP-MS (Martin, Watling & Lee 2012), or two-dimensional GC-ToF-MS (Šuklje et al. 2019) to analyse regionality effects on wines, but ICP-MS and NMR have not previously been used in combination.
Commercially available vintage wines, labelled as ‘Pinot noir’, were chosen for this study, and were either purchased from retailers and wholesalers, or donated by wineries. Four vintages (2010, 2011, 2012, and 2013) were included, limited by the availability of vintages on the market. Wines were chosen from a range of climates across three Australian states: warm South Australia (McLaren Vale, Southern Fleurieu, and Adelaide Hills), cool Victoria (Yarra Valley and Mornington Peninsula), and cooler Tasmania. The relatively cold region of Central Otago, New Zealand, was chosen as a statistical control.
The two methods used, NMR and ICP-MS, are complementary for analytical chemistry. NMR provides information on metabolites considered to provide information on berry development. ICP-MS provides information on inorganic anions (metal ions), which can be derived from the vineyard soil, as well as from subsequent vineyard and winery processes. The lab work was carried out by Drs Susanne Klein, Anna Werwein, and Christina Spartz, at the Institut Heidger in Germany, which routinely carries out such work for wineries.
For assistance with statistical analysis, I am grateful for assistance from colleagues Prof Régis Gougeon and Dr Laurence Dujourdy, at the Université de Bourgogne and AgroSup Dijon, France. We trialled a number of statistical techniques, with the main emphasis on PCA (principle component analysis). Hierarchical clustering analysis was introduced to produce dendrograms; multifactorial analysis evaluated the contribution of ICP-MS and NMR; and analysis of coinertia explored the covariance between the datasets. Multidimensional scaling and t-distributed stochastic neighbour embedding were trialled but ultimately were not used for the final analyses. All statistical analyses were conducted using GNU R.
Initially, the results were confused by several ‘statistical outlier’ samples. ICP-MS showed that these samples were high in unusual metal ions, which implied the presence of mineral deposits in the vineyards that may be atypical of their regions. Similar results were noted with NMR. When these ‘outlier’ samples were excluded from the analysis, the results became clearer. Distinct groups were evident in both the PCA and hierarchical clustering analyses. Confidence ellipses (statistical groupings) created from the PCA analyses broadly coincided with regions (by ICP-MS), and the Australian state boundaries (by NMR), but with some overlapping (see the figures below). It is worth noting that this may be partially caused by the presence in the study, of wines from different vintages (a key limitation).

The multifactorial analysis showed that ICP-MS made the highest contribution to the identification of wines in this study. NMR was partially useful, however the combination of data sources was more useful than a single data source. Confidence ellipses were also used to categorise wine by region, and the South Australian regions proved quite distinctive. The analysis of coinertia also suggested that the two datasets were not redundant.
While this was a relatively small scale (pilot) study, the results were intriguing. We hope they will provide a basis for further study, and increased using of metabolomics in the confirmation of wine regionality, thus for the detection of wine fraud. As new methods are being developed, the use of machine learning is potentially of interest (Mascellani et al. 2021; Wu et al. 2019). This has applications in most wine-producing regions, including Portugal where it would be particularly of interest for prestigious wines, such as Port (Monforte, Jacobson & Silva Ferreira 2015).
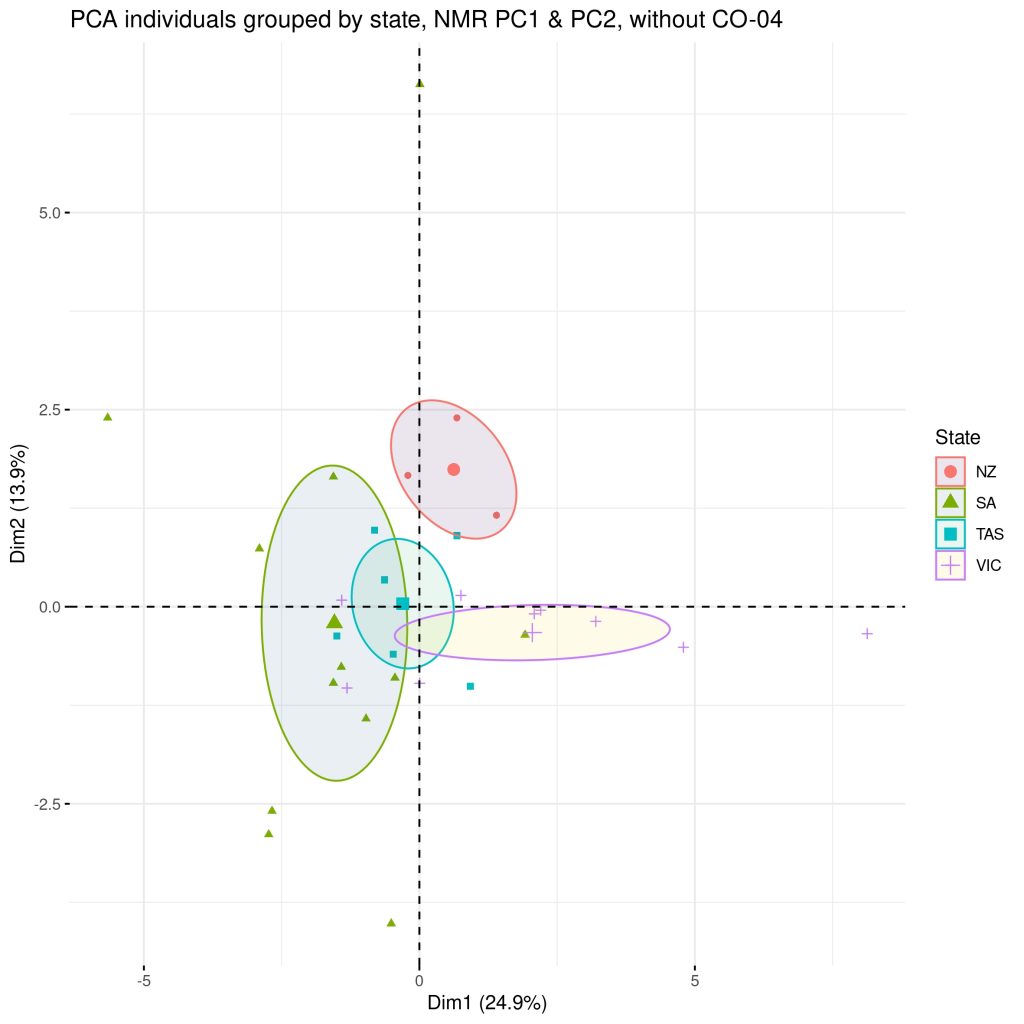
Full details of the study can be found in our paper: Duley, GP, Dujourdy, L, Klein, S, Werwein, A, Spartz, C, Gougeon, RD & Taylor, DK 2021, ‘Regionality in Australian Pinot noir wines: a study on the use of NMR and ICP-MS on commercial wines’, Food Chemistry, vol. 340. https://doi.org/10.1016/j.foodchem.2020.127906
The full paper can currently be read for free without a subscription until 22nd October 2020 at https://authors.elsevier.com/a/1bgVN16Ds1ljVq
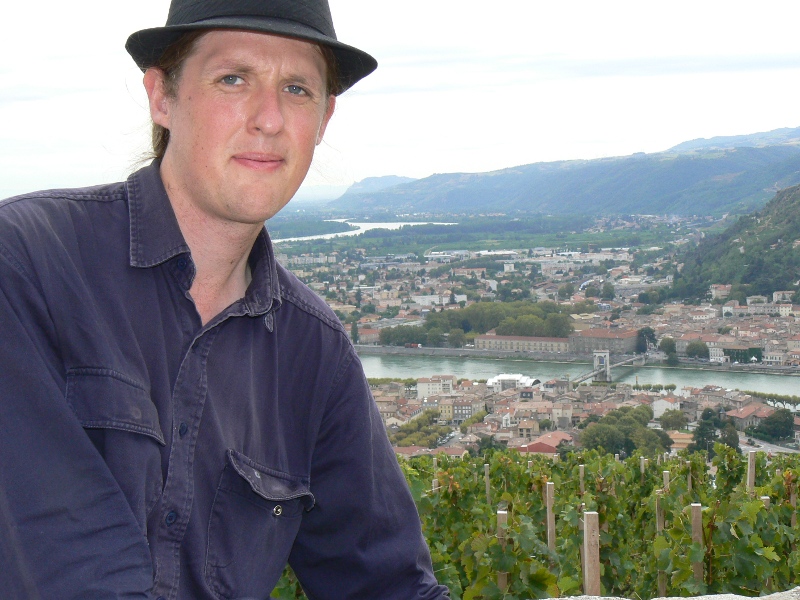
Gavin Duley recently completed a Master of Philosophy in Oenology at the University of Adelaide, Australia. His worked focussed on Pinot noir, looking both at the impact of climate change on colour in Pinot noir wines, and the use of metabolomics in determining regionality. While he currently teaches English online to university students and edits scientific papers, he is searching for an interesting PhD project. He will be presenting this work at the XIIIth ‘International Terroir Congress’, to be held online.
References
- Barnard, KN 2016, ‘The Terroir of Pinot Noir Wine in the Willamette Valley, Oregon – A Broad Analysis of Vineyard Soils, Grape Juice and Wine Chemistry’, Portland State University, Portland, OR.
- Bellomarino, SA, Conlan, XA, Parker, RM, Barnett, NW & Adams, MJ 2009, ‘Geographical classification of some Australian wines by discriminant analysis using HPLC with UV and chemiluminescence detection’, Talanta, vol. 80, no. 2, pp. 833–838.
- Martin, AE, Watling, RJ & Lee, GS 2012, ‘The multi-element determination and regional discrimination of Australian wines’, Food Chemistry, vol. 133, no. 3, pp. 1081–1089.
- Mascellani, A, Hoca, G, Babisz, M, Krska, P, Kloucek, P & Havlik, J 2021, ‘1H NMR chemometric models for classification of Czech wine type and variety’, Food Chemistry, vol. 339, p. 127852.
- Monforte, AR, Jacobson, D & Silva Ferreira, AC 2015, ‘Chemiomics: Network Reconstruction and Kinetics of Port Wine Aging’, Journal of Agricultural and Food Chemistry, vol. 63, no. 9, pp. 2576–2581.
- Pereira, L, Gomes, S, Barrias, S, Gomes, EP, Baleiras-Couto, M, Fernandes, JR & Martins-Lopes, P 2018, ‘From the Field to the Bottle—An Integrated Strategy for Wine Authenticity’, Beverages, vol. 4, no. 4, p. 71.
- Roullier-Gall, C, Boutegrabet, L, Gougeon, RD & Schmitt-Kopplin, P 2014, ‘A grape and wine chemodiversity comparison of different appellations in Burgundy: Vintage vs terroir effects’, Food Chemistry, vol. 152, pp. 100–107.
- Šuklje, K, Carlin, S, Antalick, G, Blackman, JW, Deloire, A, Vrhovsek, U & Schmidtke, LM 2019, ‘Regional Discrimination of Australian Shiraz Wine Volatome by Two-Dimensional Gas Chromatography Coupled to Time-of-Flight Mass Spectrometry’, Journal of Agricultural and Food Chemistry, vol. 67, no. 36, pp. 10273–10284.
- Wu, H, Tian, L, Chen, B, Jin, B, Tian, B, Xie, L, … Lin, G 2019, ‘Verification of imported red wine origin into China using multi isotope and elemental analyses’, Food Chemistry, vol. 301, p. 125137.